Generative AI (gen AI) is maturing at an auspicious moment for the real estate industry. Investors have mountains of both proprietary and third-party data about properties, communities, tenants, and the market itself. This information can be used to customize existing gen AI tools so that they can perform real estate–specific tasks, such as identifying opportunities for investors at lightning speed, revolutionizing building and interior design, creating marketing materials, and facilitating customer journeys while opening up new revenue streams.
Although gen AI has only recently captured the public’s imagination, AI has been fundamentally changing the way the world does business for decades. This more familiar version of AI—also known as analytical AI—is goal oriented and focused on activities such as predicting values for a future forecast or assigning categories to segment customers. It is already embedded in parts of the business world: AI-assisted forecasts, for example, have altered how investment professionals think about the future, and dynamic pricing models have changed how several industries charge for goods and services. One industry in which AI’s transformative power has been missing, however, is real estate, a historically slow adopter of new technologies.1
Gen AI represents a fresh chance for the real estate industry to learn from its past and transform itself into an industry at technology’s cutting edge. Gen AI has not replaced analytical AI; instead, its open-ended and creative nature introduces a new frontier of use cases that analytical AI does not address. Based on work by the McKinsey Global Institute (MGI), we believe that gen AI could generate $110 billion to $180 billion or more in value for the real estate industry.2
For all the hype that gen AI has received to date, many real estate organizations are finding it difficult to implement and scale use cases, and thus have not yet seen the promised value creation. This is not surprising: deriving competitive advantage from gen AI is not as simple as just deploying one of the major foundational models, and many things have to go right in an organization to make the most of the opportunity. To help companies get started, this article will explore the technology’s potential and provide examples of use cases, each of which real estate actors we work with are in some phase of exploring or implementing. The article will then describe seven pivotal, interconnected actions that real estate companies can take to realize the full value of this gen AI–fueled vision and create strategic distance from their peers.
Real estate can benefit from gen AI in a multitude of use cases
Gen AI’s strengths generally fall within one of four categories, which we dub “the four Cs.” The first is customer engagement, which can be supported by tools such as conversational chatbots that answer questions and remove doubt from customer decisions. The second is creation, in the form of tools that generate new creative content, including text and images. The third is concision: gen AI excels at synthesizing insights from unstructured data, interpreting conversations, and querying large data sources. The fourth is coding solutions, of which gen AI offers many, including interpreting, translating, and generating code.
In our own work with AI, we have seen real estate companies gain over 10 percent or more in net operating income through more efficient operating models, stronger customer experience, tenant retention, new revenue streams, and smarter asset selection. Here are five examples of how businesses can apply gen AI’s four Cs to specific real estate issues.
Sifting through mountains of leasing documentation (concision)
Gen AI can be applied to a repository of lease documents, which can be dense and filled with bespoke terminology, making it difficult for owners of many properties to sift through and find information at scale. A gen AI–powered tool can summarize key themes across the leases, such as how much rent is expected monthly or what market forces (such as local environmental, social, and governance compliance laws) could affect leases. Additionally, the tool can scan across leases for a particular parameter (for example, all leases with a rent price per square foot below a certain level) and generate tables of information. At that point, professionals can examine the information the AI tool has compiled.
Copiloting real estate interactions (concision and customer engagement)
Gen AI can be used to create a powerful copilot (a gen AI–powered bot) for a variety of real estate interactions, including managing tenant requests and lease negotiation. Simple requests from tenants, such as for routine maintenance, can prompt the copilot to directly contact a building’s maintenance staff. The copilot can identify a more complex question and flag it for a specialist at a property management company. As the specialist interacts with tenants, gen AI can observe conversations and written responses and suggest ways to improve communication. For high-stakes moments—such as a commercial lease negotiation with an office, warehouse, or retail tenant—a gen AI tool can take in all the information about a tenant, the property, and the market and craft a negotiation transcript. If communications and calls are recorded or turned to text, the copilot can monitor these interactions at scale, providing coaching while reminding specialists to refrain from using certain terms that could incite moments of risk.3
Enabling visualization and creating new revenue streams (creation and customer engagement)
Today, when a prospective office tenant looks at raw space on a tour or a potential resident views pictures of an apartment on a listing site, they see an empty unit or photos filled with someone else’s finishes and furniture. Virtual reality tours have helped, but these static, noncustomizable simulations usually only go part of the way toward showing the end user what the result could be.
Gen AI tools can help a potential tenant visualize exactly what an apartment would look like in, say, their preferred midcentury modern style or in cherrywood versus walnut finishes. This data can then be fed back into a model to predict which types of furnishings and finishes work best for different customer segments, improving prospect-to-lease conversion and shaping future capital expenditure decisions.
There can also be e-commerce tie-ins: as a prospective tenant tours a unit, an app can virtually impose a variety of couches, window trims, or kitchen appliances that match a desired design style. If the prospective resident decides to buy or lease, these choices can be ordered and set up to coincide with the move-in. The resident benefits by moving into a home that already expresses their signature style, and the brokerage or apartment company benefits by reaping revenue from cross-selling.
One large furniture retailer has launched a gen AI–powered product visualization tool that enables users to upload a photo of a room and populate it with furniture from its catalog. A variety of businesses throughout the value chain can use this capability to create new revenue streams while deepening customer loyalty.
Making faster, more precise investment decisions (concision)
Today, investment decisions are often informed through individual analysis of bespoke data pulls across sources. An investor interested in warehouses, for example, typically starts by performing a macroanalysis of markets that have attractive factors such as ports, airport locations, and high e-commerce volume. Then, they perform more granular analysis to locate areas of interest, pulling building information from local brokers or digital tools. As part of the decision-making process, the investor conducts discrete analyses to figure out how their investment hypotheses have panned out in the past.
With a gen AI tool that’s fine-tuned using internal and third-party data, an investor can simply ask, “What are the top 25 warehouse properties up for sale that I should invest in?” or, “Which malls are most likely to thrive in the future?” The tool can sort through the unstructured data—both internal (such as the performance of a company’s existing properties and the lease terms related to this performance) and third party (such as the US Census and publicly recorded, comparable sales). This multifaceted analysis can be overlaid on a list of properties for sale to identify and prioritize specific assets that are worth manual investigation.
Drawing architectural plans known to create desired outcomes (creation)
In website design, there are specific patterns and design choices known to generate e-commerce sales or higher click-through. Similarly, there are underlying design principles in the physical world that gen AI can unlock and use to draw architectural plans.
A gen AI–assisted process can introduce Internet of Things sensors and computer vision4 algorithms that collect data points on space use, such as how customers move through a store before purchase or when conference rooms are used in an office. This insight—along with outcome data about sales, customer loyalty, productivity, employee retention, or other areas—can then be fed to a gen AI tool. This information can be overlaid with spatial data about square footage, location, walls, furniture, and other architectural elements. The gen AI tool can then develop architectural plans that are optimized to create desired outcomes in a space. Human architects and designers can work from these plans to ensure art and emotion in the design, but with less guesswork over whether a space is purpose driven (illustration).
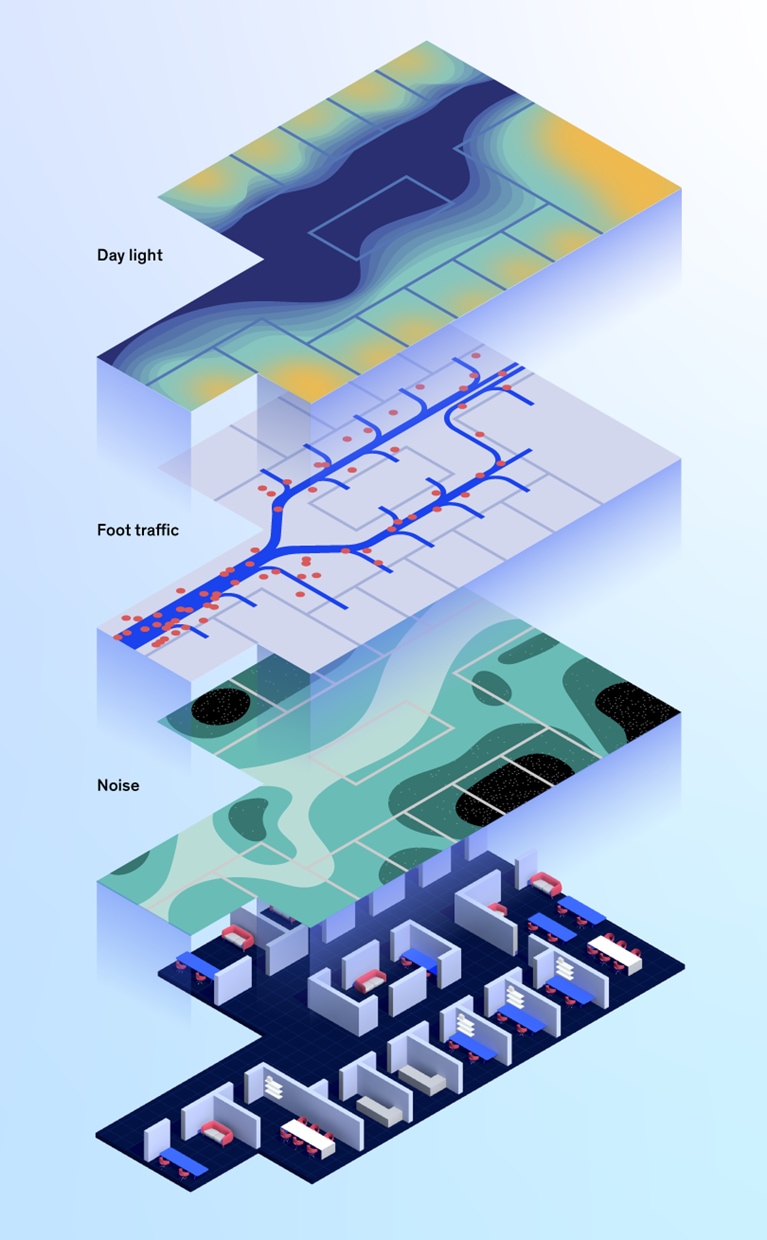
Illustration of gen AI–assisted architectural designs that show where daylight would fall on this design, how the plan would accommodate typical foot traffic patterns, and whether noise levels would distract people in the space.
Source: McKinsey research and experience with sensor and computer vision data
Seven pivotal actions real estate players can take to realize the full value of gen AI
Gen AI holds the promise of transformation, but real estate companies will have to do more than just learn how to use off-the-shelf products. Although foundational models are essential, they are just a small component of a real estate firm’s ability to realize value from gen AI.
To seize the opportunity, businesses in the real estate value chain can strive to outcompete by rewiring the way they work in the following ways.
Align the C-suite around a business-led road map tied to a specific part of the real estate value chain
CEOs who want to lead in gen AI can prioritize technology, onboard new internal capabilities, and organize for agile delivery just as top start-ups and tech-native companies do. New ways of delivering technology are essential not just to gen AI delivery but also to ensuring modernity and staying ahead of the strategic curve. Winners are willing to experiment, iterate, and self-disrupt.
That starts with having capabilities that go beyond the traditional real estate IT organization. This does not mean leaders have to welcome scores of new tech hires into their companies. Rather, it requires investing in a nimble squad of engineers and designers who are familiar with gen AI and can be directed to focus exclusively on value-adding use cases.
C-suites can start by assessing which part of the real estate value chain they occupy—such as development, operations, or investment—and considering how the journeys of tenants, employees, and other stakeholders can be reinvented. Then, they can begin redesigning roles and structures to make the alignment happen. Getting value from gen AI requires that executives be willing to question the industry’s traditional hierarchies and operating models and, most important, to accept a new technology layer throughout the organization. Gen AI requires executive-led adoption of new ways of working that will elevate the power of professionals across functions and levels.
Adopt a laser focus on data—especially new proprietary data
In the gen AI future, those with access to and control over unique, informative data will be able to generate insights that others cannot. Companies can start by thinking about what data they need—as well as what proprietary data about tenants and properties is available but not currently being collected.
It is essential not only to have the best data set but also to have it engineered the right way with the right data governance. A conversational AI tool that has been trained on a building’s past maintenance requests can efficiently respond to resident complaints. A tool trained on a real estate portfolio’s net-operating-income data can provide answers about performance that could be useful for investment decisions and for reporting to investors and internal company divisions. Internet of Things sensors and computer vision applications in office buildings, for example, can provide anonymized insights into how tenants use spaces, creating nuanced views of the built environment.5 Tenant apps and dashboards are not merely interaction channels; they themselves can become data sources. What kind of amenity space a residential tenant books, what stores a shopper in a mall browses, or what services an office tenant needs to produce an event are all valuable pieces of data that can be harnessed and structured.
It is important that this harnessing and structuring occurs in a data lakehouse controlled by the real estate firm (as opposed to by a third-party system). A variety of vendors should be able to interact with this single source of truth. This will allow real estate firms to cut data by building, tenant, or type of unit or space for their own internal uses and to be flexible enough to switch systems and applications easily. Companies should be conscious of data ownership and should make the ability to easily access and work with data a key part of vendor decision making as they design their future tech stacks.
Engineer a prompt library that gets results from foundational models in a real estate context
Foundational gen AI models are only as good as the questions (known as “prompts”) asked of them. As models are fine-tuned with data specific to real estate, it’s important to engineer a prompt library.
A prompt could say, for example, “Use the following resident history and property data to craft an initial outreach email to a resident looking to renew their lease,” followed by, “Generate a follow-up email based on their response and consider offering one of the following more-personalized concessions based on what you know about the resident: waived pet fee for two months, complimentary deep carpet cleaning, or membership to the building’s gym for six months.”
Slight edits in syntax, detail, or framing can yield meaningfully different outputs with an impact that can only be discovered in action. There is no precedent for knowing what works until it is tried. To create a playbook, a rigorous process of testing and refining to ensure questions return expected answers is essential.
Create digital tools that promote action—not just insight
Newly popular large language models fascinate the public because of how easy it can be to write prompts and receive a comprehensible response. But real estate firms should not be lulled into thinking that all gen AI outputs are intuitive or that plug-and-play point solutions deliver the full promise of gen AI. Instead, outputs often need another layer of digital tooling to be useful to an organization.
A foundational model, for example, may generate marketing copy, but it may require additional tooling to check it for grammar and brand compliance and ensure the language abides by regulations. A gen AI model may come up with insights on how customer service should be delivered by a real estate agent, but agents may need to receive prompts in a specific cadence during client interactions or explanations that clarify why a particular recommendation is important.
Design is another crucial component. Color, style, and physical-design patterns for how a user clicks are paramount in traditional apps. With a gen AI interface, it could be more important to fine-tune a conversational system’s writing style or to make sure the customer-facing avatar and its spoken tone and pitch are agreeable to the audience and encourage desired behaviors. In short, the definition of design will have to expand as a new playing field of psychology tied to interactions with algorithms and machines emerges.
A self-service portal that makes it easy for employees to access company-approved tools and learn how to use them can enable organization-wide innovation.
Invest in a modern technology stack to enable data use
A vital leg of the gen AI stool is the tech stack: the right infrastructure, feedback loops, safeguards, and integration should be built in a secure, scalable, and user-friendly way.
Gen AI requires new capabilities relative to traditional AI and machine learning, including toxicity checks (ensuring gen AI is not creating problematic content, such as words or statements that would violate relevant fair-housing or antidiscrimination laws) and guardrails against hallucination (preventing gen AI from providing false answers without sharing that the tool is uncertain). Compared with traditional data science and analytics, gen AI relies more on engineering unique tech stack elements to make it effectively operational—capabilities that real estate businesses may lack in their current IT organizations.
Real estate companies across the value chain that embrace early proofs of concept and start orienting their tech stacks in the right direction to enable use cases in the future will be in the best position to benefit. Fruitful actions include taking the time to thoughtfully link vendor systems and connect the dots on data across property management systems, customer relation management, and maintenance portals.
Adopt a new operating model that can scale as a real estate portfolio grows and diversifies
To enable a gen AI upgrade to processes for investing, leasing, and more, operating models and jobs may need to be redrawn to match the new focus points of work.
New roles and capabilities, such as prompt and data engineers who can implement foundational models, may be needed. People in existing roles, such as agents or on-site staff, may be able to hand off time-consuming tasks to gen AI tools, allowing them to focus on specialized tasks. In other cases, such as on marketing and investing teams, gen AI may evolve the discipline and create demand for new roles and skill sets. Companies need to be open to change, because the face of the IT or marketing organization will not look the same with AI tooling, even if the objectives of the business unit remain the same.
Recognize and mitigate risks unique to real estate created by gen AI
Gen AI is new, maturing, and facing open questions. There may be biases in training data that are unintentional but create outputs with real consequences. There may also be questions about the intellectual properties feeding foundational models as the legal precedent around the space evolves.6 Marketing content, for example, may emerge from an algorithm trained on unlicensed images, catching the real estate business unawares. Provider tweaks to the foundational model that underpins a real estate–specific tool could create unforeseen consequences for output quality and content, making it important to reengineer prompts.
Additionally, some use cases may not be right for gen AI in its current stage. These might include emergency response, where the stakes are too high, or leasing decisions, where it could be difficult to comply with regulations that require explanation.
Deploying gen AI in situations where investment professionals, operating teams, and tenants meet introduces new risks to working, living, and shopping environments that have to be weighed. Companies that identify risks early on and iterate to find improvements will be positioned to react effectively.
We believe that the time is right for real estate to make the leap to gen AI for two primary reasons: first, the technology has now caught up to the problems that real estate companies face and, when properly designed and executed, can provide game-changing solutions. And second, commercial real estate today faces headwinds, and these new challenges demand innovative approaches. Gen AI will not replace analytical AI—there are use cases (such as producing a rent forecast or a retention prediction) for which more traditional machine learning excels. Rather, gen AI is opening up use cases that were never before possible and are relevant to dimensions of the real estate value chain that technology did not previously touch.
But can an industry that is a reputed tech laggard overcome structural challenges, invest in underlying technology, restructure operating models, and emerge as a gen AI leader? The fact that real estate has been a laggard may actually be an advantage, because the industry did not overinvest in previously available but now-outdated technology as some other industries did.7 The learning curve may be steeper for those who have not yet invested in technology and data strategies, but we believe gen AI’s insights, speed, and transformational power for the real estate industry are worth the climb. Data—and use of that data to create strategic distance—are the new terrain on which businesses will compete for investor dollars, tenants, buyers, and longevity.
The idea of getting started can be daunting, but we urge executives to start simply. Our technology professionals advocate for a “2x2” approach: identify two use cases that can launch a company into taking ownership of data, deliver measurable impact quickly, and build excitement; and identify two use cases that are more aspirational, will fundamentally change the business, and take more time to deliver. This approach encourages companies to push the technology toward its full potential.
There is no question that the real estate industry is changing. The question is which companies will answer that call of change, adapt, and embrace a new, digitally powered future.