Amid the intense competition and cost-cutting confronting telcos, early evidence suggests that generative AI (gen AI) could be the catalyst to reignite growth after a decade of stagnation. But will it be a groundbreaking differentiator or simply table stakes?
Already the technology is on track to become a new norm in the industry. Most telco leaders we surveyed1 say they are developing gen AI solutions that range from pilots to full-scale deployments, and leading telcos such as AT&T, SK Telecom, and Vodafone have made much-publicized early gen AI commitments and launched trials. Some telcos around the world have started to experience significant double-digit percentage impact from this technology. One European telco recently increased conversion rates for marketing campaigns by 40 percent while reducing costs by using gen AI to personalize content. A Latin American telco increased call center agent productivity by 25 percent and improved the quality of its customer experience by enhancing agent skills and knowledge with gen-AI-driven recommendations.
Most impressive is that these telcos deployed the models in just weeks—the first went live in two weeks, and the second in five. For an industry with a mixed track record for capitalizing on new technologies and legacy systems that slow innovation, these early results and deployment times illustrate the potentially transformative power of gen AI.
These aren’t one-offs. Pretrained models that can be fine-tuned in days for use cases are readily available, enabling organizations to bring proofs-of-concept to life with minimal up-front investment, achieve impact out of the gate, and scale their efforts. Our experience working with clients indicates the potential for telcos to achieve significant EBITDA impact with gen AI. In some cases, estimates indicate returns on incremental margins increasing 3 to 4 percentage points in two years, and as much as 8 to 10 percentage points in five years, by enhancing customer revenue through improved customer life cycle management and decisively reducing costs across all domains.
However, while nearly all of the 130 telcos we surveyed are doing something with gen AI, our survey findings suggest a palpable sense of caution and skepticism in the industry. More than 85 percent of the executives surveyed are cautious to attribute more than 20 percent revenue or cost savings impact by domain, with the greatest enthusiasm for a radical transformation in customer service (Exhibit 1).
This blend of optimism and restraint highlights the critical juncture the industry faces. Seizing the gen AI opportunity to differentiate services and achieve sustainable growth will require the hidebound industry to embrace innovation, exploration, and agility at an unprecedented level and move from decoupled AI efforts to a holistic, AI-native telco.
The chance for telcos to make this change has never been more accessible. The industry has struggled these last ten-plus years to achieve the potential of “traditional” AI, given the complexity and legacy processes involved. In addition to the significant impact gen AI can bring to bear with entirely new use cases and applications, its ability to learn from vast amounts of diverse data and interact in near-human-like ways may be the tipping point for accelerating broader AI programs and the building blocks that enable them, fueling company-wide transformations.
Furthermore, the imperative for such change has never been greater. Because gen AI democratizes access to powerful capabilities, any telco—a small operator or large incumbent—can reshape customer expectations and its organizational efficiency. In doing so, they can potentially narrow previously unassailable competitive advantages and overturn long-standing barriers to growth. Those at the forefront of this movement stand to position themselves to regain growth faster and capture a more significant share of the nearly $100 billion in incremental value (Exhibit 2). That is in addition to the $140 billion to $180 billion in productivity gains that gen AI will create in the industry above what could be unlocked by traditional AI.
How can telco leaders use the technology to drive AI transformations and unlock new value? What challenges do they face? And what will it take to succeed? This article offers insights into these critical questions, drawing extensively from our research, industry survey, and first-hand experience implementing these technologies.
Gen AI today in the telco industry
Gen AI represents the latest advance in AI, and it may arguably be one of the most important. The technology’s ability to analyze more and different types of data such as code, images, and text, and to create new content, enables new levels of personalization, performance, and customer engagement. With today’s capabilities, many use cases are already possible across network operations, customer service, marketing and sales, IT, and support functions.
These use cases can both enhance existing AI capabilities (through the inclusion of new unstructured data sources) and provide new sources of value (through gen AI and in combination with traditional AI solutions) to deliver significant impact across all key domains. Customer service and marketing and sales currently make up the largest share of total impact (Exhibit 3).
Examples of such use cases based on early pilots include the following:
- In customer service, where the technology can vastly improve customer experience, increase agent productivity, and enable fully digital interactions. A Latin American telco is enhancing its customer service AI chatbots to improve agent support, a move it anticipates will reduce costs by 15 to 20 percent. The telco also is using gen AI to summarize voice and written client interactions in nearly a dozen use cases, with the expectation that it can reduce associated costs by up to 80 percent.
- In marketing and sales, where gen AI enables hyperpersonalization, deeper customer insights, and faster content generation. A European telco is using the technology to identify new sales leads from customer calls, with its pilot project achieving a more than 10 percent conversion rate. The company can now also create personalized messages and visual media to target individual customer microsegments. To do this, the telco feeds a gen AI model standard marketing messages, customer data (including household details, type of phone they use, and where they live), and cognitive biases (for example, whether the customer would be more receptive to messaging that evokes scarcity, such as a limited time offer, or emphasizes authority, such as endorsements, awards, and industry experience).
- In network operations, gen AI can optimize technology configurations, enhance labor productivity, extract customer insights from social media, and improve inventory and network planning and management through the ability to mine unstructured data. One large telco is using the technology to accelerate network mapping by analyzing and structuring data about network components, including specifications and maintenance information, from supplier contracts. This will enable the telco to more accurately assess component compatibility, maintenance requirements, and more—an effort anticipated to improve operational planning and optimize capital productivity.
- In IT, where the technology can accelerate software migrations and development, gen AI offers telcos a path to reduce their mounting technical debt and enable capabilities previously deferred because of time and resource constraints. Organizations are applying gen AI to streamline the entire software life cycle, from documenting how a new product, feature, or service will be perceived by end users to generating and scanning code for vulnerabilities before launch. One McKinsey study found that software developers can complete coding tasks up to twice as fast with gen AI.
- In support functions, where gen AI will reduce the costs associated with back-office operations and improve employee productivity. A European telco uses the technology in a number of way, including: shortening procurement analysis and negotiation strategy insights from weeks to a few hours, reducing recruiting costs with automated screening and recommendations, improving employee productivity using internal gen chatbots and copilots, and automating most internal content generation. Combined, the company anticipates these efforts will improve employee productivity by 30 percent.
New sources of value may also emerge from turning internal uses cases into new products for their customers. For example, a customer care solution may be offered on demand to small business customers seeking ways to improve their own call center’s productivity and service.
Would you like to learn more about our Technology, Media & Telecommunications Practice?
Telco leaders’ view ahead: Real change and real challenges
In the wake of their initial successes, business leaders we surveyed say they plan to maintain or double their budgets for gen AI in the next year and invest in more than 50 dedicated full-time employees to pursue their gen AI ambitions effectively. More than half are already scaling up use cases.
Moreover, survey findings indicate that the technology also had a knock-on effect across all AI initiatives. Compared to responses from McKinsey’s 2022 digital twin survey, we see a 30-percentage-point increase in business leaders who want to invest in and focus more on data and analytics.
However, despite the magnitude of the opportunity and the level of interest (and need), our survey found few that follow the kind of holistic approach required to succeed at scale. Only about one-third of telco leaders said they have a capability-building plan for employees on gen AI or are investing in change management efforts—two core building blocks for building a culture of innovation and the test-and-learn mindset. A similar share said gen AI has yet to be treated as an organizational priority, and that proponents of the technology often encounter difficulties in justifying use cases—a clear signal that much of the push has come from the bottom, not the top, and that more work is needed to elevate gen AI to a CEO-led priority. Moreover, finding appropriate talent and obtaining quality data remain significant challenges for telcos, although confidence about solving these rose among surveyed leaders this year as compared to last.
Finally, the survey findings suggest that gen AI has already begun to influence long-standing market dynamics. While European telecom operators have traditionally lagged in AI and technology transformations, survey findings indicate that they are pulling ahead of those in North America in their adoption of gen AI, especially in areas such as network operations (71 percent compared to 58 percent) and IT (67 percent compared to 55 percent). This shift may be a result of greater maturity in managing data privacy. Small and large operators report similar views on where to prioritize, focusing on customer service and IT in similar measure, suggesting the possibility of new competitive pressures emerging for incumbents (Exhibit 4).
The building blocks for a successful gen AI journey
In order to achieve the above-mentioned impact, organizations will need to move away from the labyrinth of proofs-of-concept and scale the technology. As with any digital or AI initiative, we find there are no shortcuts in doing this. The same core building blocks are necessary, namely (1) a business-led roadmap, (2) the right talent, (3) an operating model for scale, (4) technology built for speed and innovation, (5) quality data that is easily accessible and managed in a responsible and accountable way, and (6) change management to ensure adoption and scaling. These are fundamental pillars in effectively scaling use cases and capturing sustainable impact from gen AI in the journey toward an AI-native telco.
However, while the same holistic approach is required, gen AI’s unique capabilities—its ability to surface new insights from seemingly unrelated data, its reliance on large language models from third-party vendors, and its transformative impact on roles and work—present new challenges that will require greater agility and additional oversight. Next, we outline key differences and provide recommendations on how telcos can best tackle them.
Strategy: Determine when to build, buy, or fine-tune solutions
As with any AI initiative, leaders will need to align on vision, value, and road map, assessing both risks and opportunities, and communicating guidelines for use across the organization. In building a road map, telco leaders will face a choice: use a commercial, off-the-shelf solution if one exists, fine-tune existing large language models with internal data, or build and train a new foundation model from scratch (what we refer to as the “taker,” “shaper,” and “maker” approach, respectively).
Each is suitable for different use cases and has its own costs, requiring leaders to develop not only a clear vision and strategy for which use cases to pursue, but also how. One mistake we see some telcos making is building common gen AI solutions from scratch––a content generator or call summarization solution for example—when there are nearly a dozen out-of-the-box options on the market today from gen AI start-ups or SaaS vendors injecting gen AI capabilities into existing solutions. Only one-third of surveyed telco leaders say they buy products off-the-shelf, suggesting that many telcos continue to embrace a do-it-yourself model. This move is likely to slow innovation and distract talent from more differentiating use cases, as it has in the past with other technologies.
Instead, leaders should strongly consider partnering with gen AI solution providers and enterprise software vendors for solutions that aren’t very complex or telco specific. This is particularly critical in instances where any delays in implementation will put them at a disadvantage against competitors already leveraging these services. The handful of solutions leaders can concentrate on shaping or making themselves should enable them to differentiate their offerings or address a strategic business priority, such as delivering the best service or network coverage, and drive sustained economic impact. To do this, one CIO at a large telco is bringing together business leaders across all key domains to assess hundreds of potential use cases and build a road map for determining when to build, buy, or fine-tune models, and for prioritizing resources and building momentum with early successes.
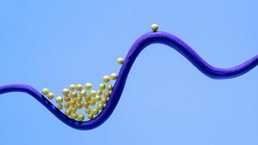
The AI-native telco: Radical transformation to thrive in turbulent times
Talent: Upskill and expand internal expertise to innovate with gen AI
The speed of innovation that is now possible with gen AI puts new pressure on telcos accustomed to outsourcing tech talent to build in-house AI expertise. Consider the experiences of two telcos—one that continued offshoring and outsourcing tech talent and one that created a dedicated AI team of ten data scientists and engineers. In the time the first telco took to draft requirements for outsourcing gen AI use-case development, the second built and deployed four gen AI solutions.
While this new technology democratizes AI by requiring fewer highly specialized data scientists to build the models, it requires new skills, such as gen AI prompt engineering, which may sometimes be a separate skill embedded within traditional roles. It also requires significantly more data engineers and subject matter experts who understand what data to collect and how, and who can oversee daily quality reviews as new forms of data are generated and consumed by these systems, including user queries, responses, and feedback.
Capturing the full potential will also require significant upskilling of existing staff—everyone from data scientists to business leaders—on gen AI, including the risks of uploading proprietary data into third-party language models. Some telcos are setting up internal certification and university-led training programs to ensure their teams have the right skills and capabilities to innovate and execute with the technology. For instance, the large telco created a badging system to identify gen-AI-ready employees who have completed the company’s sessions on use, risk, and effective prompting techniques given by its AI, legal, and risk experts. Following certification, users participate in weekly discussion groups to stay abreast of changes and discuss their successes and challenges. McKinsey research has found such efforts improve the quality of prompts.
Operating model: Orchestrate efforts enterprise-wide
A significant portion of implemented gen AI solutions can be adapted and reused in multiple use cases. A gen AI chatbot developed to improve agent productivity, for example, can be repurposed with additional fine-tuning or data to answer frequently asked questions by new employees or provide IT support. An off-the-shelf content generation system for drafting sales proposals may also streamline the development of marketing and business plans.
As a result, we’re beginning to see telcos adopt more centralized decision making around gen AI development. This shift includes a greater emphasis on adopting reusable services and self-service components, an evolution of key functions, such as risk, FinOps, and transformation offices, to be more focused on gen AI, and the creation of “control towers” that can oversee all gen AI investments and development efforts. In practice, that can mean, for example, prioritizing the use-case pipeline, identifying opportunities for reusability, setting key performance indicators to measure and track impact at the level of both use case and enterprise, and managing suppliers and risk. The European telco’s control tower evaluates the effects of its gen AI transformation based on three dimensions—financial impact, user adoption, and model performance—and aggregates the data in dashboards that enable the company’s top executives to keep tabs on the organization’s progress. Similarly, a Latin American telco uses a control tower to consolidate and standardize supplier contracts, tracking key metrics such as scope, duration, and renewal to compare providers more easily, identify potential redundancies, and reduce the manual work of digitizing content.
Technology: Create a blueprint for reusability, innovation, and excellence
Organizations will also need a technology blueprint that enables reusability. For instance, the blueprint should include a framework for determining which large language models to use and when (commercial or open-source models for example, or those that support hybrid workloads). And it should lay out how to scale a pilot, for example to extend a pilot that serves 100 call agents to serve more than 10,000 agents with the same latency and cost profile. The blueprint should also have a framework for determining which gen AI capabilities can be turned into ready-to-use modules to be plugged into different use cases. One large telco, for example, has begun to identify and develop components designed to fetch product data from a large dataset and generate content from it that could be reused by data science teams across domains such as customer service, network operations, and sales and marketing.
With new gen AI research and capabilities being announced weekly and sometimes daily, technology teams will also need a dedicated gen AI innovation lab to keep abreast of industry changes and test emerging solutions. For example, one large telco’s chief data and analytics officer recruited PhD graduates from universities to staff a gen AI innovation lab and build bespoke solutions ahead of the market to gain a competitive edge.
Once new models are deployed, telcos will need to monitor model outputs daily to ensure quality and accuracy do not waver as models learn and adapt their responses based on user queries and feedback. Large language model operations (LLMOps) is an emerging practice that aims to streamline the daily management and monitoring of gen AI models. A key component of LLMOps is a dedicated operations team to oversee all deployed gen AI models, continuously monitoring for issues and rapidly adapting solutions when needed, just as a network operations team might do for network performance. Organizations can start small now and build capability in this area as the field of LLMOps develops. For example, the European telco started by assigning three data scientists to monitor their handful of deployed models and plans to expand the team as more models are deployed.
Data: Capture everything, especially unstructured data, and ensure responsible use
One of gen AI’s superpowers is its ability to uncover connections in seemingly unrelated data sets, which has implications for how organizations choose to collect and measure data, and how they manage it to ensure responsible use.
Data collection: Telcos will need to think more broadly about data collection, mapping more data, setting up pipelines for unstructured data, and creating synthetic data to evaluate outputs. A US telco, for instance, has reached beyond its traditional datasets in its work to develop a customer service agent copilot that will reduce average resolution times by 40 percent across more than 1 million annual chats. As part of their work, the company’s data scientists gather institutional knowledge from agent emails and interactions to enable the chatbot to learn from real situations and challenges, and offer detailed descriptions of how to resolve specific issues. The team also creates synthetic data using a large language model to create sample customer questions and answers, with agents reviewing the outputs for accuracy.
Responsible use: These types of more sophisticated data strategies and tactics come with new regulatory, intellectual property, and data privacy concerns. Risks abound in this new era, particularly with customer insights, recommendations, and network optimizations being analyzed and generated by third-party large language models and open-source environments. To address the novel risks, telcos need to expand their data governance programs to address unstructured data. For example, one multinational telco hardware provider created a robust data access process to validate what data can be used in gen AI use cases. Data owners along with legal and security experts work together to validate each use case based on several criteria including the criticality of the data to the business (data that is deemed of high importance cannot be input into commercial large language models); the end users (some users cannot access certain data assets); and the risks if the gen AI solution gives an incorrect answer. The team manages the process in an agile manner using a simple Microsoft Power App to manage and automate the workflow across teams, and conducts monthly forums to review the process and develop improvements. The organization has reviewed more than 200 use cases, rejecting a number due to intellectual property and other risks, to ensure responsible use for the company.
Change management: Ensure adoption and scaling are CEO-led
Every role, including everyone from network technicians to HR professionals, will be impacted by gen AI, making vital the need for leaders to begin preparing their employees now to capture the full value of this transformative technology. With many employees already using the technology in their personal lives, organizations will need to consider how to help them learn to apply the technology in a professional context, upskilling and reskilling staff at scale. Such work can be made easier using gen AI, for example to develop and deliver customized and adaptive training programs, and even to onboard employees.
For example, another European telco saw firsthand the importance of change management and upskilling when it created a gen-AI-driven knowledge “expert” that helped agents get answers to customer questions more quickly. The initial pilot, which didn’t include any process changes or employee education, realized just a 5 percent improvement in productivity. As the organization prepared to scale the solution, leaders dedicated 90 percent of the budget to agent training and change management processes, which facilitated the adoption of the solution and resulted in more than 30 percent productivity improvement. The telco also used gen AI to create upskilling programs and provide agents with personalized recommendations for improvement once the solution was rolled out.
Even though so many companies have already achieved real cost savings and revenue improvements with gen AI, these are still the early days of the technology. In the next five years, emerging capabilities—including significant improvements in natural language understanding, advances in human-like reasoning across multiple topics, and availability of real-time solutions with increased accuracy and fewer hallucinations—should unlock even more exciting opportunities beyond the basic improvements seen today.
Combined, these gen AI capabilities will enable telcos to redefine industry standards and set themselves apart in the market. For example, network operations could be enhanced and quality standards radically recast with AI copilots that evaluate images from technicians, provide accurate recommendations for remedies, and automatically initiate interventions or work orders. In sales, cognitive copilots could conduct sentiment analysis on customer calls in real time and guide sales representatives on how best to respond, profoundly altering sales strategies, customer engagement, and overall sales outcomes. Customer service channels using cognitive chatbots could seamlessly answer complex queries in real time while taking into account privacy and fairness concerns, thereby revolutionizing efficiency while offering customers a human-like experience. Across the enterprise, greater efficiency and productivity could emerge as domain-specific solutions endowed with an organization’s institutional knowledge power an unprecedented wave of automation and AI-driven decision making.
The sudden rise of gen AI has brought the dream of the AI-native telco significantly closer to becoming a reality. With it comes the opportunity for telcos to reverse their recent stagnant fortunes and usher in a new era of growth and innovation. The journey will not be easy, however. To answer the call of gen AI, telcos will need to quickly adopt a culture of innovation and experimentation enabled by the core building blocks shared in this article, one they have previously struggled to build and maintain. With the technology moving so rapidly, those operators that embrace it now are likeliest to create a significant lead that will be difficult for others to follow.